Spatial Resolution Improvement with Unsupervised Estimation of Non-Ideal Focal Spot Effect for Computed Tomography
AAPM ePoster Library. Zhang Z. 07/12/20; 302025; PO-GeP-I-193
Topic: Cone-beam CT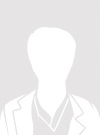
Zhicheng Zhang
Contributions
Contributions
Abstract
Poster Number: PO-GeP-I-193
Abstract ID: 52109
Spatial Resolution Improvement with Unsupervised Estimation of Non-Ideal Focal Spot Effect for Computed Tomography
Z Zhang*, X Li, L Yu, X Liang, L Xing, Stanford Univ School of Medicine, Stanford, CA
Imaging General ePoster
Category: Scientific:Imaging Physics:Cone-beam CT:Reconstruction
Purpose:With other conditions fixed, the smaller focal spot size of X-Ray, the higher spatial resolution of computed tomography (CT) image, however, the smaller the X-Ray output per unit time of the X-Ray source, the higher the cost and the lower the temporal resolution. Therefore, how to use a low-cost X-Ray source to obtain high spatial resolution CT image which is used to be available only from a high-cost CT with a high-quality X-Ray source.
Methods:We introduced the mathematical idea for image deblurring and employed regularization by denoising (RED) as the regularization for the inverse problem of image deblurring, and then utilized a 5-layer convolutional neural network as the denoiser of choice. We transferred the inverse problem into a neural network and designed a cascaded convolutional neural network with five repeating subnetwork structures, all the relative parameters in each subnetwork were shared to minimize the number of parameters of the entire network to facilitate network training. After collecting the sinogram Y to be reconstructed, CT image X was reconstructed firstly. To train the well-designed neural network in an unsupervised fashion, a simulated sinogram Ys from CT image X with a random focal spot size was fed as input and X was treated as the ground truth in each training iteration.
Results:On the Catphan700 phantom, the proposed method can improve the MTF50% by 7.5% and MTF10% by 3.5%. In the Ham experiment directly tested with the well-trained model on the Catphan700 phantom, the proposed method can tell the fine bone structure inside the ham bone more clearly, see visual results in Fig. 3 in the supporting document.
Conclusion:The experimental results demonstrate that the proposed method can improve the CT image resolution with only simulated signogram derived from the sinogram to be reconstructed as the training data in an unsupervised fashion.
Taxonomy:IM- CT: Image Reconstruction
Keywords: CT,deconvolution,high-resolution imaging,
Abstract ID: 52109
Spatial Resolution Improvement with Unsupervised Estimation of Non-Ideal Focal Spot Effect for Computed Tomography
Z Zhang*, X Li, L Yu, X Liang, L Xing, Stanford Univ School of Medicine, Stanford, CA
Imaging General ePoster
Category: Scientific:Imaging Physics:Cone-beam CT:Reconstruction
Purpose:With other conditions fixed, the smaller focal spot size of X-Ray, the higher spatial resolution of computed tomography (CT) image, however, the smaller the X-Ray output per unit time of the X-Ray source, the higher the cost and the lower the temporal resolution. Therefore, how to use a low-cost X-Ray source to obtain high spatial resolution CT image which is used to be available only from a high-cost CT with a high-quality X-Ray source.
Methods:We introduced the mathematical idea for image deblurring and employed regularization by denoising (RED) as the regularization for the inverse problem of image deblurring, and then utilized a 5-layer convolutional neural network as the denoiser of choice. We transferred the inverse problem into a neural network and designed a cascaded convolutional neural network with five repeating subnetwork structures, all the relative parameters in each subnetwork were shared to minimize the number of parameters of the entire network to facilitate network training. After collecting the sinogram Y to be reconstructed, CT image X was reconstructed firstly. To train the well-designed neural network in an unsupervised fashion, a simulated sinogram Ys from CT image X with a random focal spot size was fed as input and X was treated as the ground truth in each training iteration.
Results:On the Catphan700 phantom, the proposed method can improve the MTF50% by 7.5% and MTF10% by 3.5%. In the Ham experiment directly tested with the well-trained model on the Catphan700 phantom, the proposed method can tell the fine bone structure inside the ham bone more clearly, see visual results in Fig. 3 in the supporting document.
Conclusion:The experimental results demonstrate that the proposed method can improve the CT image resolution with only simulated signogram derived from the sinogram to be reconstructed as the training data in an unsupervised fashion.
Taxonomy:IM- CT: Image Reconstruction
Keywords: CT,deconvolution,high-resolution imaging,
Poster Number: PO-GeP-I-193
Abstract ID: 52109
Spatial Resolution Improvement with Unsupervised Estimation of Non-Ideal Focal Spot Effect for Computed Tomography
Z Zhang*, X Li, L Yu, X Liang, L Xing, Stanford Univ School of Medicine, Stanford, CA
Imaging General ePoster
Category: Scientific:Imaging Physics:Cone-beam CT:Reconstruction
Purpose:With other conditions fixed, the smaller focal spot size of X-Ray, the higher spatial resolution of computed tomography (CT) image, however, the smaller the X-Ray output per unit time of the X-Ray source, the higher the cost and the lower the temporal resolution. Therefore, how to use a low-cost X-Ray source to obtain high spatial resolution CT image which is used to be available only from a high-cost CT with a high-quality X-Ray source.
Methods:We introduced the mathematical idea for image deblurring and employed regularization by denoising (RED) as the regularization for the inverse problem of image deblurring, and then utilized a 5-layer convolutional neural network as the denoiser of choice. We transferred the inverse problem into a neural network and designed a cascaded convolutional neural network with five repeating subnetwork structures, all the relative parameters in each subnetwork were shared to minimize the number of parameters of the entire network to facilitate network training. After collecting the sinogram Y to be reconstructed, CT image X was reconstructed firstly. To train the well-designed neural network in an unsupervised fashion, a simulated sinogram Ys from CT image X with a random focal spot size was fed as input and X was treated as the ground truth in each training iteration.
Results:On the Catphan700 phantom, the proposed method can improve the MTF50% by 7.5% and MTF10% by 3.5%. In the Ham experiment directly tested with the well-trained model on the Catphan700 phantom, the proposed method can tell the fine bone structure inside the ham bone more clearly, see visual results in Fig. 3 in the supporting document.
Conclusion:The experimental results demonstrate that the proposed method can improve the CT image resolution with only simulated signogram derived from the sinogram to be reconstructed as the training data in an unsupervised fashion.
Taxonomy:IM- CT: Image Reconstruction
Keywords: CT,deconvolution,high-resolution imaging,
Abstract ID: 52109
Spatial Resolution Improvement with Unsupervised Estimation of Non-Ideal Focal Spot Effect for Computed Tomography
Z Zhang*, X Li, L Yu, X Liang, L Xing, Stanford Univ School of Medicine, Stanford, CA
Imaging General ePoster
Category: Scientific:Imaging Physics:Cone-beam CT:Reconstruction
Purpose:With other conditions fixed, the smaller focal spot size of X-Ray, the higher spatial resolution of computed tomography (CT) image, however, the smaller the X-Ray output per unit time of the X-Ray source, the higher the cost and the lower the temporal resolution. Therefore, how to use a low-cost X-Ray source to obtain high spatial resolution CT image which is used to be available only from a high-cost CT with a high-quality X-Ray source.
Methods:We introduced the mathematical idea for image deblurring and employed regularization by denoising (RED) as the regularization for the inverse problem of image deblurring, and then utilized a 5-layer convolutional neural network as the denoiser of choice. We transferred the inverse problem into a neural network and designed a cascaded convolutional neural network with five repeating subnetwork structures, all the relative parameters in each subnetwork were shared to minimize the number of parameters of the entire network to facilitate network training. After collecting the sinogram Y to be reconstructed, CT image X was reconstructed firstly. To train the well-designed neural network in an unsupervised fashion, a simulated sinogram Ys from CT image X with a random focal spot size was fed as input and X was treated as the ground truth in each training iteration.
Results:On the Catphan700 phantom, the proposed method can improve the MTF50% by 7.5% and MTF10% by 3.5%. In the Ham experiment directly tested with the well-trained model on the Catphan700 phantom, the proposed method can tell the fine bone structure inside the ham bone more clearly, see visual results in Fig. 3 in the supporting document.
Conclusion:The experimental results demonstrate that the proposed method can improve the CT image resolution with only simulated signogram derived from the sinogram to be reconstructed as the training data in an unsupervised fashion.
Taxonomy:IM- CT: Image Reconstruction
Keywords: CT,deconvolution,high-resolution imaging,
{{ help_message }}
{{filter}}